Detection of grids on nuclear fuel set images
Detekce distančních mřížek na palivovém souboru
diplomová práce (OBHÁJENO)
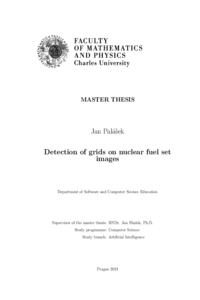
Zobrazit/ otevřít
Trvalý odkaz
http://hdl.handle.net/20.500.11956/127314Identifikátory
SIS: 219219
Kolekce
- Kvalifikační práce [11242]
Autor
Vedoucí práce
Oponent práce
Šikudová, Elena
Fakulta / součást
Matematicko-fyzikální fakulta
Obor
Umělá inteligence
Katedra / ústav / klinika
Katedra softwaru a výuky informatiky
Datum obhajoby
22. 6. 2021
Nakladatel
Univerzita Karlova, Matematicko-fyzikální fakultaJazyk
Angličtina
Známka
Výborně
Klíčová slova (česky)
jaderné palivo|segmentace obrazu|mřížkaKlíčová slova (anglicky)
nuclear fuel|image segmentation|gridVizuální kontrola palivových souborů je nezbytná pro identifikaci anomálního chování spojeného s jejich stavem a budoucím použitím. Jedním z možných nálezů jsou cizí před- měty zachycené na distanční mřížce, které mohou za provozu narušit pokrytí palivových proutků. Cílem této práce je přesně segmentovat z obrázku distanční mřížku, což je du- ální úloha k detekci cízích předmětů, a automatizovat tak proces vizuální kontroly v této oblasti. Vytvořili jsme nové datasety pokrývající typické problémy paliva. Pro provedení segmentace jsme využili neuronové sítě. Zvýšili jsme výkon algoritmu použitím datové augmentace a doménově specifickým zpracováním výstupu. Změřili jsme výkonnost al- goritmu pomocí nově zavedené metriky Line Distance, která počítá velikost maximální nejisté oblasti mezi skutečným a předpovězeným přechodem mezi distančními mřížkami a pruty. V experimentech jsme našli nejlepší hyperparametry a dosáhli velmi dobrých vý- sledků. Překonali jsme našeho předchůdce tím, že naše Line Distance metrika byla třikrát nižší. 1
Visual inspection of fuel assemblies is necessary to identify potential anomalies in their behaviour associated with their condition and their future usage. One of the possible find- ings are foreign objects caught on the fuel spacer grid which can disrupt the cladding of fuel rods during the operation. The goal of this thesis is to accurately segment the spacer grid from an image, which is a task dual to the foreign object detection, and therefore to automate visual inspection process in this area. We created new datasets covering typical problems appearing on the fuel assembly. To perform the segmentation, we em- ployed neural networks. We increased performance by data augmentation techniques and domain-specific output post-processing. We also measured the algorithm's performance by a newly introduced Line Distance metric, computing the size of the maximum un- certain area between the actual and the predicted transition between grids and rods. In the experiments, we found the best hyperparameters and reached very good results, outperforming our predecessor's algorithm by having three times lower Line Distance metric. 1