Detecting Misleading Features in Data Visualization
Rozpoznání zavádějících prvků ve vizualizacích dat
bachelor thesis (DEFENDED)
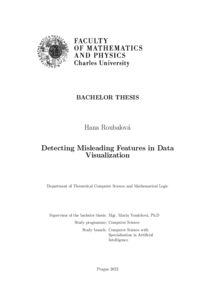
View/ Open
Permanent link
http://hdl.handle.net/20.500.11956/184416Identifiers
Study Information System: 249976
Collections
- Kvalifikační práce [11242]
Author
Advisor
Referee
Červíčková, Věra
Faculty / Institute
Faculty of Mathematics and Physics
Discipline
Computer Science with specialisation in Artificial Intelligence
Department
Department of Theoretical Computer Science and Mathematical Logic
Date of defense
7. 9. 2023
Publisher
Univerzita Karlova, Matematicko-fyzikální fakultaLanguage
English
Grade
Excellent
Keywords (Czech)
vizualizace dat|dezinformace|barvoslepost|vnímání barev|vada barevného viděníKeywords (English)
data visualization|misinformation|colorblindness|color perception|color vision deficiencyTato práce se zabývá detekcí zavádějících prvků ve vizualizacích dat. Teoretická část práce seznamuje s různými typy běžně se vyskytujících zavádějích prvků a ukazuje je na konkrétních příkladech. Implementační část práce pak s pomocí analýzy různých algoritmů představuje aplikaci určenou k rozpoznání nevhodných grafů pro jedince s bar- voslepostí. Práce ukazuje, jak může software zjednodušit detekci zavádějících prvků pro běžného uživatele. Zároveň zvyšuje povědomí o tomto problému a představuje možnosti prevence proti negativním důsledkům zavádějících vizualizací. 1
This thesis explores the identification and detection of misleading elements in data visu- alizations. The theoretical portion focuses on understanding various types of misleading features commonly encountered in scientific figures and recognizing them. The imple- mentation introduces an application designed to detect colorblind-unfriendly graphs with the analysis of various algorithms. The thesis raises awareness about misleading visual- izations and demonstrates how software can simplify the detection of misleading features for the everyday user. This thesis highlights the importance of addressing misleading features in data visualizations and introduces an application to assist in their detection. The study advances our understanding of this field and offers insights into reducing the negative effects of misleading data visualizations. 1