Finding errors and inconsistencies in the CorefUD coreference dataset
Hledání chyb a nekonzistencí v koreferenčním datasetu CorefUD
bakalářská práce (OBHÁJENO)
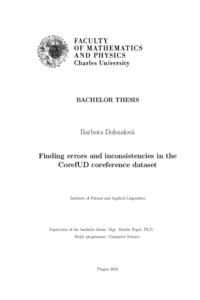
Zobrazit/ otevřít
Trvalý odkaz
http://hdl.handle.net/20.500.11956/192872Identifikátory
SIS: 255447
Kolekce
- Kvalifikační práce [11326]
Autor
Vedoucí práce
Oponent práce
Novák, Michal
Fakulta / součást
Matematicko-fyzikální fakulta
Obor
Informatika se specializací Obecná informatika
Katedra / ústav / klinika
Ústav formální a aplikované lingvistiky
Datum obhajoby
5. 9. 2024
Nakladatel
Univerzita Karlova, Matematicko-fyzikální fakultaJazyk
Angličtina
Známka
Výborně
Klíčová slova (česky)
koreference|detekce chyb v anotaci|CorefUDKlíčová slova (anglicky)
coreference|annotation error detection|CorefUDProjekt CorefUD se snaží harmonizovat anotaci koreference napříč různými jazyky, po vzoru iniciativy Universal Dependencies. Od roku 2022 je CorefUD dataset využíván v CRAC Shared Task on Coreference Resolution, soutěži, ve které je cílem zúčastněných automaticky koreferenci anotovat. Jelikož jsou predikce zúčastněných systémů veřejně dostupné, rozhodli jsme se otestovat s jejich pomocí následující domněnku: Pokud se většina predikcí shodne, že by zlatá data měla být anotovaná jinak, potenciálně to ukazuje na chybu právě ve zlatých datech. Abychom ji ověřili, naprogramujeme PluCorAED, systém na detekci anotačních chyb, který klasifikuje chyby, které predikce udělaly, a posčítá je. Následně provedeme analýzu výsledků, abychom zjistili, pro které typy chyb je tento přístup vhodný. Nakonec shrneme chyby, které jsme našli a opravili v CorefUDu - Některé z oprav již byly zakomponovány do nejnovější verze.
The CorefUD project attempts to harmonise coreference annotation accross different languages, in the spirit of the Universal Dependencies initiative. Since 2022 it is also used in the CRAC Shared Task on Coreference Resolution, where participants try to annotate the data automatically. As the submission predictions are publicly available, we decided to test the following hypothesis: If most of the predictions agree that the gold annotation should be different, it might indicate an error in the gold data. To verify this, we build PluCorAED, an annotation error detection system, which classifies errors the submissions made and aggregates them. Then we analyse the results to see which types of errors this approach might be suitable for. Finally, we give an overview of the errors we have found and corrected in CorefUD. Some of the corrections have already been incorporated into a new release.