Logistická regrese s misklasifikovanou odezvou
Logistic regression with misclassified response
diplomová práce (OBHÁJENO)
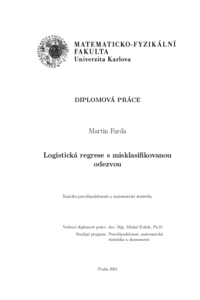
Zobrazit/ otevřít
Trvalý odkaz
http://hdl.handle.net/20.500.11956/193118Identifikátory
SIS: 270305
Kolekce
- Kvalifikační práce [11325]
Autor
Vedoucí práce
Oponent práce
Antoch, Jaromír
Fakulta / součást
Matematicko-fyzikální fakulta
Obor
Pravděpodobnost, matematická statistika a ekonometrie
Katedra / ústav / klinika
Katedra pravděpodobnosti a matematické statistiky
Datum obhajoby
6. 9. 2024
Nakladatel
Univerzita Karlova, Matematicko-fyzikální fakultaJazyk
Čeština
Známka
Výborně
Klíčová slova (česky)
logistická regrese|misklasifikovaná odezva|EM algoritmusKlíčová slova (anglicky)
logistic regression|misclassified response|EM algorithmCílem práce je podrobně představit metody analýzy logistické regrese s misklasifi- kovanou odezvou a porovnat je na příkladech. První kapitola poskytuje stručný úvod k logistické regresi a EM algoritmu. Druhá kapitola popisuje metody analýzy při znalosti skutečné senzitivity a specificity. Třetí kapitola se zaměřuje na situaci, kdy skutečná sen- zitivita a specificita nejsou známé, a stručně uvádí možné přístupy k této problematice. Čtvrtá kapitola se podrobně věnuje situaci kdy senzitivita a specificita nejsou známé, ale je k dispozici validační skupina. Kapitola řeší jak situaci kdy senzitivita a specificita jsou konstanty tak situaci kdy mohou záviset na vysvětlujících proměnných. Součástí kapitoly jsou odvození pozorované informační matice a intervalů spolehlivosti. Závěrečná kapitola se věnuje porovnání metod prostřednictvím simulační studie.
The aim of this thesis is to present in detail the methods of logistic regression analysis with a misclassified response and to compare them with examples. The first chapter provides a brief introduction to logistic regression and the EM algorithm. The second chapter describes the analysis methods while knowing the true sensitivity and specificity. The third chapter focuses on the situation where the true sensitivity and specificity are not known and briefly introduces possible approaches to this problem. The fourth chap- ter discusses in detail the situation where sensitivity and specificity are unknown but a validation group is available. The chapter addresses both the situation where sensitivity and specificity are constants and the situation where they may depend on explanatory variables. The chapter includes derivations of the observed information matrix and con- fidence intervals. The final chapter is devoted to a comparison of the methods through a simulation study.