Material picker: Material recognition in images using machine learning
Material picker: Rozpoznávání materiálů v obraze pomocí strojového učení
diploma thesis (DEFENDED)
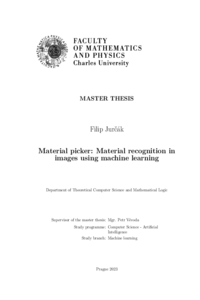
View/ Open
Permanent link
http://hdl.handle.net/20.500.11956/188481Identifiers
Study Information System: 234932
Collections
- Kvalifikační práce [11325]
Author
Advisor
Referee
Šikudová, Elena
Faculty / Institute
Faculty of Mathematics and Physics
Discipline
Computer Science - Artificial Intelligence
Department
Department of Software and Computer Science Education
Date of defense
13. 2. 2024
Publisher
Univerzita Karlova, Matematicko-fyzikální fakultaLanguage
English
Grade
Very good
Keywords (Czech)
strojové učení|hluboké učení|počítačová grafika|inverzní renderování|segmentace materiálů|rozpoznávání materiálůKeywords (English)
machine learning|deep learning|computer graphics|inverse rendering|material segmentation|material recognitionProces nastavování vlastností materiálu pro realistický vzhled po vyrenderování je obvykle únavný a často vyžaduje pečlivě nadobudnutou zručnost pro jemné doladění parametrů, protože různé kombinace těchto parametrů mohou produkovat různě vy- padající materiály. Pro zjednodušení tohoto procesu představujeme řešení problému přenosu textur vytvořením nástroje obsahujícího několik hlubokých neuronových sítí. Tyto sítě následně představují řešení pro inverzní renderování a segmentaci materiálu předpovídáním vnitřních charakteristik scény, jako je difuzní a lesklé albedo, povrchové normály, lesk, pohledový vektor, texturové souřadnice a segmentace, toto vše z jednoho snímku. Umělci mohou následně použít tyto odvozené vlastnosti ve svých reprezentací 3D scény a zkrátit tak čas potřebný k iteraci několika nápadů návrhů. Abychom tyto sítě trénovali, vygenerovali jsme vysoce kvalitní datovou sadu značné velikosti pomocí fyzicky založených technik, abychom zajistili dobrou generalizaci na obrázky z reálného světa. 1
The process of setting material properties for realistic appearance after rendering is usually tiresome and often requires carefully crafted skill for fine-tuning the parameters, as different combinations of these parameters can produce different-looking materials. To simplify this process, we introduce a solution to the texture transfer problem by creating a pipeline containing several deep neural networks. These networks subsequently represent solutions to inverse rendering and material segmentation by predicting intrinsic scene characteristics, like diffuse and specular albedo, surface normals, glossiness, view vector, texture coordinates, and segmentation, all from a single image. Artists can subsequently plug these inferenced properties inside their 3D scene representations and thus reduce the time needed to iterate over several design ideas. To train these networks, we generated high-quality dataset of substantial size using physically-based techniques to ensure good generalization on real-world images. 1