Věty o univerzalitě a konzistenci neuronových sítí
Universality and consistency theorems for neural networks
diploma thesis (DEFENDED)
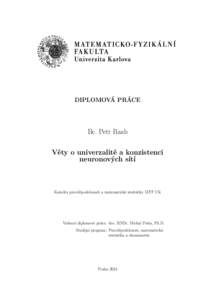
View/ Open
Permanent link
http://hdl.handle.net/20.500.11956/190572Identifiers
Study Information System: 269723
Collections
- Kvalifikační práce [11325]
Author
Advisor
Referee
Hlubinka, Daniel
Faculty / Institute
Faculty of Mathematics and Physics
Discipline
Probability, Mathematical Statistics and Econometrics with specialisation in Econometrics
Department
Department of Probability and Mathematical Statistics
Date of defense
10. 6. 2024
Publisher
Univerzita Karlova, Matematicko-fyzikální fakultaLanguage
Czech
Grade
Very good
Keywords (Czech)
věty o univerzalitě|věty o konzistenci|neuronové sítě|hluboké učení|asymptotické chování|sítový odhadKeywords (English)
universality theorems|consistency theorems|neural networks|deep learning|asymptotic behavior|sieve estimatorPráce se zabývá neuronovými sítěmi a modelem hlubokého učení, kdy se autor snaží na neuronové sítě dívat jako na statistický model podobný zobecněným lineárním modelům. Po představení tohoto modelu a zavedení značení se práce věnuje schopnosti neuronových sítí aproximovat spojité funkce, kdy je předveden důkaz věty o univerzalitě. Následně jsou zkoumány asymptotické vlastnosti neuronových sítí, pomocí sítového odhadu je také dokázána jejich konzistence a asymptotická normalita. Právě tyto dvě vlastnosti jsou objektem zkoumání v simulační studii na generovaných datech. 1
The work deals with neural networks and deep learning models, where the author attempts to view neural networks as a statistical model similar to generalized linear models. After introducing this model and introducing the notation, the work focuses on the ability of neural networks to approximate continuous functions, with a proof of the universality theorem presented. Subsequently, the asymptotic properties of neural networks are examined, and using network estimation, their consistency and asymptotic normality are also proven. These two properties are precisely the subject of investigation in a simulation study on generated data. 1