Využití kombinovaných sekvenčních a strukturních vlastností pro predikci protein-ligand vazebných míst
Using combined sequence and structural features to predict protein-ligand binding sites
diploma thesis (DEFENDED)
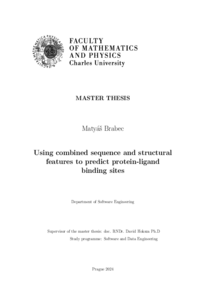
View/ Open
Permanent link
http://hdl.handle.net/20.500.11956/190729Identifiers
Study Information System: 267838
Collections
- Kvalifikační práce [11326]
Author
Advisor
Referee
Škoda, Petr
Faculty / Institute
Faculty of Mathematics and Physics
Discipline
Computer Science - Software and Data Engineering
Department
Department of Software Engineering
Date of defense
11. 6. 2024
Publisher
Univerzita Karlova, Matematicko-fyzikální fakultaLanguage
Czech
Grade
Excellent
Keywords (Czech)
bioinformatika|proteinová sekvence|proteinová struktura|strojové učeníKeywords (English)
bioinforamtics|protein sequence|protein structure|machine learningInterakce proteinů s různými molekulami jsou klíčové pro jejich funkci a následně pro celkové fungování organismů. Studium těchto interakcí je důležité v mnoha vědních obo- rech, včetně medicíny, kde má zásadní význam pro vývoj léků. Klíčovým typem těchto interakcí je vazba mezi proteinem a ligandem a významným cílem bioinformatiky je vy- vinout spolehlivé modely pro predikci těchto vazebných míst. Nedávný nárůst databazí proteinových struktur v kombinaci s výkonem moderních GPU umožnil vývoj mnoha modelů strojového učení. Zejména protein language modely, inspirované svými protějšky ve zpracování přirozeného jazyka, se úspěšně uplatňují v napříč bioinformatikou. V této práci jsme použili model proteinového jazyka pro predikci vazebných míst a snažili jsme se zvýšit jeho výkonnost začleněním různých trojrozměrných vlastností proteinů. 1
Protein interactions with various molecules are crucial for their function and, con- sequently, for the overall functioning of organisms. Studying these interactions is im- portant across many scientific fields, including medicine, where it is essential for drug development. Protein-ligand binding is a key type of these interactions, and a significant goal in bioinformatics is to develop reliable models for binding site prediction. The re- cent surge in the collection of protein structures, combined with the immense power of modern GPUs, has allowed the development of many machine-learning models. Notably protein language models, inspired by their counterparts in natural language processing, have been successfully applied throughout bioinformatics. In this thesis, we fine-tuned a protein language model for binding site prediction and sought to enhance its performance by incorporating various three-dimensional features of proteins. 1