Problém nejmenších čtverců s maticí, která je částečně hustá a částečně řídká
Solving linear least squares problem with sparse-dense system matrix
bachelor thesis (DEFENDED)
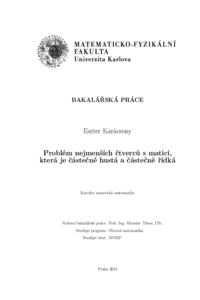
View/ Open
Permanent link
http://hdl.handle.net/20.500.11956/191744Identifiers
Study Information System: 251619
Collections
- Kvalifikační práce [11326]
Author
Advisor
Referee
Hnětynková, Iveta
Faculty / Institute
Faculty of Mathematics and Physics
Discipline
General Mathematics
Department
Department of Numerical Mathematics
Date of defense
21. 6. 2024
Publisher
Univerzita Karlova, Matematicko-fyzikální fakultaLanguage
Czech
Grade
Very good
Keywords (Czech)
problém nejmenších čtvreců|Choleského faktorizace|metoda sdružených gradientů|předpodmínění|matice částečně hustá a částečně řídkáKeywords (English)
least squares problem|Cholesky factorization|conjugate gradients|preconditioning|sparse-dense matrixTato práce se zabývá metodou nejmenších čtverců s maticí, která je částečně hustá a částečně řídká. V první části práce je popsaná metoda nejmenších čtverců a její základní vlastnosti. Poté jsou uvedené teoretické základy, na kterých jsou založeny postupy jak ře- šit soustavy rovnic s maticí částečně hustou a částečně řídkou pomocí metody nejmenších čtverců. Zvolený teoretický postup je demonstrován odvozením algoritmu CGLS s před- podmíněním. V experimentech je postup ověřen aplikací metody CGLS s předpodmíně- ním na testovací matice, které odpovídají zadání a tedy jsou částečně husté a částečně řídké. V těchto experimentech nás zajímala například změna trvání výpočtu a změna počtu iterací se zvyšujícím se počtem hustých řádků v matici. Výsledky jsou znázorněny i graficky. 1
This thesis deals with the method of least squares with matrices that are partly dense and partly sparse. The first part of the thesis describes the method of least squares and its basic properties. Then theoretical foundations of how to proceed with the solution of a system of equations with a sparse-dense matrix using the method of least squares are described. In particular, the approach of the thesis leads to the derivation of the CGLS algorithm with preconditioning. In the experiments, the preconditioned CGLS method is applied to test matrices that are partly dense and partly sparse. For example, changes in computational time and related changes in iteration counts depending on the number of additional dense rows in the matrices are investigated. The results are illustrated by several plots. 1