Transformer-Based Approaches to Research Paper Retrieval: A Benchmark Analysis with Application
Metody na Bázi Transformeru pro Vyhledávání Vědeckých Článků: Srovnávací Analýza s Aplikací
bachelor thesis (DEFENDED)
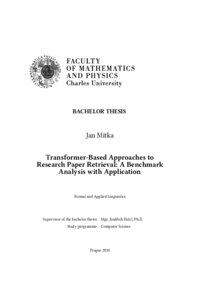
View/ Open
Permanent link
http://hdl.handle.net/20.500.11956/192940Identifiers
Study Information System: 250549
Collections
- Kvalifikační práce [11326]
Author
Advisor
Referee
Hajič, Jan
Faculty / Institute
Faculty of Mathematics and Physics
Discipline
Computer Science with specialisation in Computer Graphics, Vision and Game Development
Department
Institute of Formal and Applied Linguistics
Date of defense
5. 9. 2024
Publisher
Univerzita Karlova, Matematicko-fyzikální fakultaLanguage
English
Grade
Excellent
Keywords (Czech)
vyhledávání informací|Transformer Modely|reprezentace dokumentůKeywords (English)
Information Retrieval|Transformer Models|Document RepresentationSe zvětšujícími se databázemi roster poptávka po efektivních vyhledávacích algoritmech. Tato bakalářská práce zkoumá vyhledávání vědeckých článků pomocí modelů založených na Transformeru: BERT, SciBERT, RoBERTa a MPNet, a také specializovaných modelů: SPECTER, SciNCL a tři Sentence Transformery. Analyzujeme každý model a porovnáváme jejich výkon na testovacích datasetech z SciRepEvalu. Sentence Transformer s architekturou MPNet dosahuje nejlepších výsledků v 7 z 9 testů, přičemž překonává i modely specializované na reprezentaci dokumentů. Naše jednoduchá vyhledávací aplikace slouží jako ukázka možnosti volby modelu pro vyhledávání.
As databases expand, the demand for effective information retrieval algorithms increases. This thesis investigates the research paper retrieval using Transformer-based models: BERT, SciBERT, RoBERTa, and MPNet, and fine-tuned models: SPECTER, SciNCL, and three Sentence Transformers. We analyze each model and compare their performance on SciRepEval benchmark datasets. The Sentence Transformer with MPNet architecture achieves the best performance in 7 out of 9 tasks, outperforming even the document representation fine-tuned models. Our simple search application showcases the option for users to choose the model for searches.