Kauzálne usudzovanie a klasická ekonometria
Causal inference and classical econometrics
Kauzální usuzování a klasická ekonometrie
diploma thesis (DEFENDED)
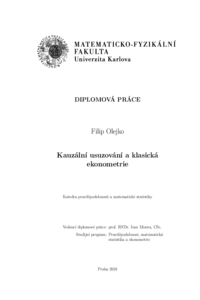
View/ Open
Permanent link
http://hdl.handle.net/20.500.11956/192963Identifiers
Study Information System: 271676
Collections
- Kvalifikační práce [11326]
Author
Advisor
Referee
Hudecová, Šárka
Faculty / Institute
Faculty of Mathematics and Physics
Discipline
Probability, Mathematical Statistics and Econometrics
Department
Department of Probability and Mathematical Statistics
Date of defense
5. 9. 2024
Publisher
Univerzita Karlova, Matematicko-fyzikální fakultaLanguage
Slovak
Grade
Good
Keywords (Czech)
Kauzálne usudzovanie|Model potenciálnych výsledkov|Inštrumentálne premennéKeywords (English)
Causal inference|Potential outcomes model|Instrumental variablesDiplomová práca poskytuje základný náhľad na teóriu používanú pri kauzálnom usud- zovaní. Zameriava sa pritom na model potenciálnych výsledkov a použitie usmernených acyklických grafov (DAGs). Tiež prezentuje jednu z klasických metód používanú v ekono- metrii, ktorá využíva inštrumentálne premenné a dvojstupňový odhad pomocou metódy najmenších štvorcov. Táto metóda je daná do kontextu so situáciou, kedy dochádza k zastieraniu a identifikácia kauzálnych vzťahov s použitím väčšiny klasických metód zly- háva. Vo vhodných situáciach potom z pohľadu modelu potenciálnych výsledkov prezen- tuje predpoklady potrebné pre kauzálne usudzovanie. Nakoniec v simulačnej štúdií pre vybrané situácie, kedy dochádza k zastieraniu, demonštruje použitie metódy používajúcej inštrumentálne premenné. V celej práci sa zdôrazňuje dôležitosť uvádzaných predpokla- dov potrebných pre vyvodenie platných záverov o kauzalite.
The thesis attempts to provide a basic insight into the theory used in causal infer- ence. In this regard, it focuses on the potential outcomes model and the use of directed acyclic graphs (DAGs). It also presents one of the classical methods used in economet- rics, which uses instrumental variables and two-stage estimation using the least squares method. This method is put in the context of a situation where there is confounding and the identification of causal relationships using more conventional methods therefore fails. In suitable situations, the assumptions necessary for causal inference from the per- spective of the potential outcomes model are presented. Finally, for selected situations where confounding occurs, the simulation study demostrates the use of a method using instrumental variables. Throughout the thesis, the importance of the stated assumptions needed to draw valid inferences about causality is emphasized.