Consistency of S-weighted estimators in panel data models
Konzistence S-vážených odhadů v modelech panelových dat
diploma thesis (DEFENDED)
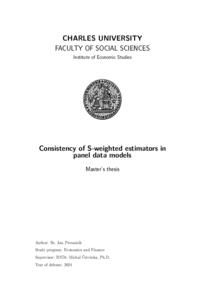
View/ Open
Permanent link
http://hdl.handle.net/20.500.11956/194805Identifiers
Study Information System: 237285
Collections
- Kvalifikační práce [18159]
Author
Advisor
Referee
Hanus, Luboš
Faculty / Institute
Faculty of Social Sciences
Discipline
Economics and Finance
Department
Institute of Economic Studies
Date of defense
18. 9. 2024
Publisher
Univerzita Karlova, Fakulta sociálních vědLanguage
English
Grade
Very good
Keywords (Czech)
S-weighted estimator, robust statistics, consistency, panel dataKeywords (English)
S-weighted estimator, robust statistics, consistency, panel dataTato práce se zaměřuje na S-vážený odhad a jeho výkon na kontaminovaných datech. První část práce shrnuje historický kontext, poskytuje základní orientaci v oblasti robustní statistiky a sumarizuje existující literaturu o S-váženém odhadu. V simulační studii provedené v jazyce Matlab porovnáváme kvalitu odhadů pořízených pomocí S-váženého odhadu s metodou LWS a S-odhadem. Výsledky ukazují, že v nízkých úrovních kontaminace je účinnost S-váženého odhadu stejná s metodou LWS. Kontaminace vyšší než 10 % způsobuje významně vyšší střední kvadratickou odchylku u S-váženého odhadu. Závěrečná část práce se věnuje jednoduché implementaci S-váženého odhadu v jazyce Python.
The thesis focuses on the S-weighted estimator and its performance on contaminated data. The first part summarizes the historical background, providing a basic orientation in the field of robust statistics and reviewing the existing literature on S-weighted estimator. In a simulation study performed in Matlab, the estimator's performance is compared with that of LWS and S- estimator. The results show that S-weighted estimator achieves the same efficiency as LWS in lower contamination levels. Contamination exceeding 10% causes significantly higher mean squared error of the S-weighted estimates. The last part of the thesis focuses on developing a simple implementation of the estimator in Python.