Isotonic Regression in Sobolev Spaces
Izotonická regrese v Sobolevových prostorech
diploma thesis (DEFENDED)
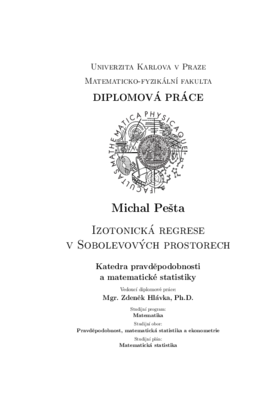
View/ Open
Permanent link
http://hdl.handle.net/20.500.11956/4483Identifiers
Study Information System: 42177
Collections
- Kvalifikační práce [11327]
Author
Advisor
Referee
Dostál, Petr
Faculty / Institute
Faculty of Mathematics and Physics
Discipline
Probability, mathematical statistics and econometrics
Department
Department of Probability and Mathematical Statistics
Date of defense
18. 5. 2006
Publisher
Univerzita Karlova, Matematicko-fyzikální fakultaLanguage
English
Grade
Excellent
Uvažme třídu neparametrických odhadů pro regresní modely založené na metodě nejmenších čtverců přes množiny dostatečně hladkých funkcí. Nejmenší čtverce dovolují uložení dodatečného omezení, izotonie, na neparametrické regresní odhady a jejich následné testování. Odhady probíhají přes koule funkcí, které jsou prvky vhodných Sobolevových prostorů. Sobolevovy prostory jsou speciální typ Hilbertových prostorů, které umožňují projekci vzhledem k nejmenší čtvercům. Hilbertovskost nám umožňuje dělat projekci a tedy rozložit prostor do navzájem kolmých doplňků. Pak převedeme problém hledání nejlépe aproximující funkce v prostoru nekonečné dimenze na konečně-dimenzionální optimalizační problém. Dokážeme, že koule funkcí Sobolevových prostorech je omezená a má omezené i derivace vyššího řádu. To nám dovoluje odhadovat přes bohatou množinu funkcí s dostatečně malou metrickou entropií a použít zákony velkých čísel a centrální limitní věty.
We propose a class of nonparametric estimators for the regression models based on least squares over the sets of sufficiently smooth functions. Least squares permit the imposition of additional constraint-isotonia-on nonparametric regression estimation and testing of this constraint. The estimation takes place over the balls of functions which are elements of a suitable Sobolev space-special types of Hilbert spaces that facilitate calculation of the least squares projection. The Hilbertness is allowing us to take projections and hence to decompose spaces into mutually orthogonal complements. Then we transform the problem of searching for the best fitting function in an infinite dimensional space into a finite dimensional optimization problem. We prove that the balls of functions in Sobolev space are bounded and have bounded higher order derivatives. It permits us to estimate over rich set of functions with sufficiently low metric entropy and apply Laws of Large Numbers and Central Limit Theorems.