Cross-lingual transfer for the annotation of the SynSemClass ontology
Mezijazykový transfer pro anotaci SynSemClass ontologie
bakalářská práce (OBHÁJENO)
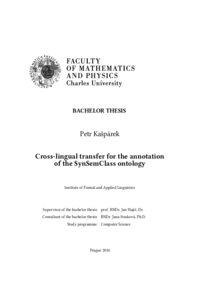
Zobrazit/ otevřít
Trvalý odkaz
http://hdl.handle.net/20.500.11956/192066Identifikátory
SIS: 256636
Kolekce
- Kvalifikační práce [11326]
Autor
Vedoucí práce
Konzultant práce
Straková, Jana
Oponent práce
Štěpánek, Jan
Fakulta / součást
Matematicko-fyzikální fakulta
Obor
Informatika se specializací Umělá inteligence
Katedra / ústav / klinika
Ústav formální a aplikované lingvistiky
Datum obhajoby
28. 6. 2024
Nakladatel
Univerzita Karlova, Matematicko-fyzikální fakultaJazyk
Angličtina
Známka
Výborně
Klíčová slova (česky)
annotation projection|zero-shot cross-lingual transfer|ontologie|vícejazyčné zpracování přirozeného jazyka|lexikální sémantikaKlíčová slova (anglicky)
annotation projection|zero-shot cross-lingual transfer|ontology|multilingual natural language processing|lexical semanticsTato pra ́ce porovna ́va ́ dva pr ̌ı 'stupy k automaticke ́ pr ̌edanotaci se ́manticky ́ch tr ̌ı ́d sloves ve ve ̌ta ́ch za u ́c ̌elem pr ̌ida ́nı ́ nove ́ho jazyka do ontologie SynSemClass. Oba pr ̌ı 'stupy vycha ́zejı ́ z vı ́cejazyc ̌ne ́ho deep learning klasifikac ̌nı ́ho modelu, ktery ́ byl fine-tunovany ́ na jiz ̌ anotovany ́ch anglicky ́ch, c ̌esky ́ch a ne ̌mecky ́ch datech z ontologie. Prvnı ́, vı ́ce tradic ̌nı ́, pr ̌ı 'stup je annotation projection. Pouz ̌ı ́va ́ paralelnı ́ korpus a vy 's ̌e zmı ́ne ̌ny ́ model k vytvor ̌enı ́ predikcı ́ na zdrojove ́m jazyce, ktery ́ je jiz ̌ obsaz ̌en v ontologii, a tyto predikce projektuje na cı ́lovy ́ jazyk pomocı ́ automaticke ́ho word alignmentu. Druhy ́ pr ̌ı 'stup, zero-shot cross-lingual transfer, pr ̌edpokla ́da ́, z ̌e vı ́cejazykove ́ schopnosti deep learning modelu jsou dostatec ̌ne ́ a z ̌e mu ̊z ̌eme vytvor ̌it kvalitnı ́ predikce pr ̌ı ́mo na cı ́love ́m jazyce, i kdyz ̌ model nebyl nikdy tre ́nova ́n pro danou u ́lohu na dane ́m cı ́love ́m jazyce. Pro u ́c ̌ely vyhodnocenı ́ ruc ̌ne ̌ vytva ́r ̌ı ́me a anotujeme maly ́ korejsky ́ dataset za u ́c ̌elem otestova ́nı ́ vy 'sledku ̊ na jazyce, ktery ́ se vy ́znamne ̌ lis ̌ı ́ od anglic ̌tiny, c ̌es ̌tiny a ne ̌mc ̌iny. Dospı ́va ́me k za ́ve ̌ru, z ̌e zero-shot transfer vykazuje vy ́razne ̌ leps...
This work compares two approaches to automatic preannotation of semantic class to verbs in a sentence for the purpose of adding a new language to the SynSemClass ontology. Both approaches rely on a multilingual deep learning classification model fine-tuned on already annotated English, Czech and German data of the ontology. The first, more classical, approach is annotation projection. It uses a parallel corpus and the aforementioned model to make predictions on a source language already present in the ontology and projects the predictions onto the target language using automated word alignment. The second approach, zero-shot cross-lingual transfer, assumes that the multilingual properties of the underlying model are sufficient and that we can make reasonable predictions directly on the target language, even though the model was never trained for that specific task on the specific target language. For the purpose of evaluation, we manually build and annotate a small Korean language dataset to test the performance on a language significantly different from English, Czech and German. We conclude that the zero-shot approach performs notably better than the alignment approach (p < 0.005) obtaining 0.54 both in recall and precision, compared to 0.37 and 0.41 in recall and precision respectively of the alignment...